Dynamic Classifier фрезерование Cuanto De Produccion Определения De Talles
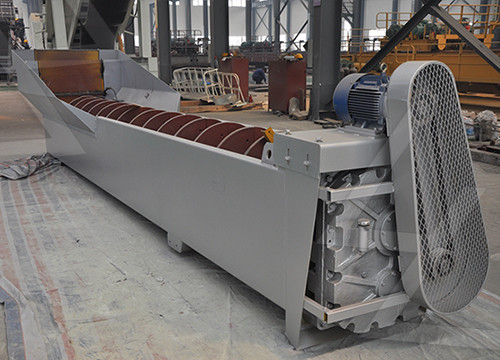
Dynamic selection of classifiers—A comprehensive review
2014年11月1日 We review the main methods of dynamic selection of classifiers. • A taxonomy for the methods of dynamic selection of classifiers is proposed. • We examine the significance of the reported results on dynamic selection. • The performance of dynamic

Preprocessed dynamic classifier ensemble selection for
2021年2月1日 The proposal of combining preprocessing and Dynamic Ensemble Selection, which is the basis of research carried out in this work, was presented in [53]. Pinagé et al. proposed a concept drift detection method based on dynamic classifier

A framework for dynamic classifier selection oriented by
2018年4月1日 This stone describes a framework for Dynamic Classifier Selection (DCS) whose novelty resides in its use of features that address the difficulty posed by the classification problem in terms of orienting both pool generation and classifier selection.

Dynamic classifier selection Information Fusion ACM
An updated taxonomy of Dynamic Selection techniques is proposed.A review of the state-of-the-art dynamic selection techniques is presented.Empirical comparison between 18 dynamic selection techniques is conducted.We discuss about the recent findings and

Research on a dynamic full Bayesian classifier for time
2021年5月16日 The dynamic full Bayesian classifier is optimized by splitting the smooth parameters into intervals, optimizing the parameters by constructing a smoothing parameter configuration tree (or forest), then selecting and averaging the classifiers. The dynamic

A framework for dynamic classifier selection oriented by
2018年4月1日 This stone describes a framework for Dynamic Classifier Selection (DCS) whose novelty resides in its use of features that address the difficulty posed by the classification problem in terms of orienting both pool generation and classifier selection.

A drift detection method based on dynamic classifier
2019年10月11日 Gama and Castillo ( 2004) claim that a significant classification error rate increase suggests a drift in the class distribution. In order to detect this type of drift, our method is inspired by drift detectors based on error monitoring such as the Drift
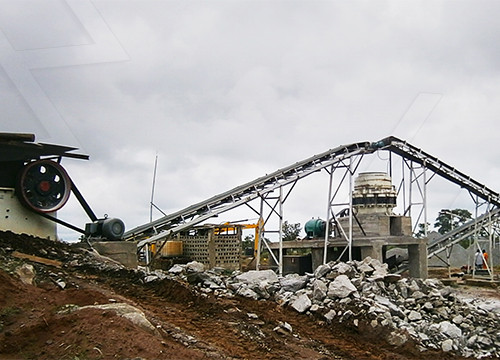
Dynamic classifier selection Information Fusion ACM
One of the most promising MCS approaches is Dynamic Selection (DS), in which the base classifiers are selected on the fly, according to each new sample to be classified. This stone provides a review of the DS techniques proposed in the literature from a theoretical and
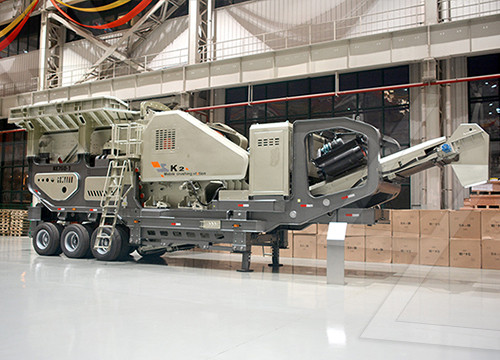
Dynamic selection of classifiers—A comprehensive review
2014年11月1日 Abstract. This work presents a literature review of multiple classifier systems based on the dynamic selection of classifiers. First, it briefly reviews some basic concepts and definitions related to such a classification approach and then it presents
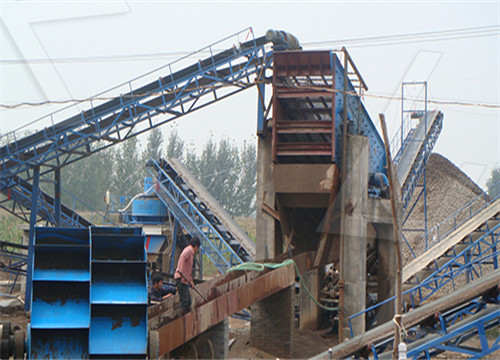
Preprocessed dynamic classifier ensemble selection for
2021年2月1日 The proposal of combining preprocessing and Dynamic Ensemble Selection, which is the basis of research carried out in this work, was presented in [53]. Pinagé et al. proposed a concept drift detection method based on dynamic classifier selection [54]. We may also consider the following des strategies based on oracle

A framework for dynamic classifier selection oriented by
2018年4月1日 This stone describes a framework for Dynamic Classifier Selection (DCS) whose novelty resides in its use of features that address the difficulty posed by the classification problem in terms of orienting both pool generation and classifier selection. A. S. Britto Jr. received M.Sc. degree in Industrial Informatics from the Centro Federal de

Dynamic classifier selection: Recent advances and
2018年5月1日 In this , we present an updated taxonomy of dynamic classifier and ensemble selection techniques, taking into account the following three aspects: (1) The selection approach, which considers, whether a single classifier is selected (this is known as Dynamic Classifier Selection (DCS)) or an ensemble is selected (this for its part is known

[1809.01628] Online local pool generation for dynamic
2018年9月6日 Dynamic Classifier Selection (DCS) techniques have difficulty in selecting the most competent classifier in a pool, even when its presence is assured. Since the DCS techniques rely only on local data to estimate a classifier's competence, the manner in which the pool is generated could affect the choice of the best classifier for a given sample.

A drift detection method based on dynamic classifier
2019年10月11日 The new unknown incoming examples that fall outside this global decision boundary are assumed as drifts or novelties; (2) DbDDM compares a dissimilarity prediction to a classifier prediction (assumed as true label) to monitor the pseudo prequential error to detect drifts by statistical tests.

Adapting dynamic classifier selection for concept drift
2018年8月15日 Our experimental results show that DCS is capable of adapting to different concept drift scenarios by selecting the most promising classifier/ensemble for each test instance, regardless of the concept used to train the selected classifiers. In addition, we show that the DCS performs well in most cases, and requires almost no parameter tuning.

From dynamic classifier selection to dynamic ensemble
2008年5月1日 We note that most dynamic classifier selection schemes use the concept of classifier accuracy on a defined neighborhood or region, such as the local accuracy A Priori or A Posteriori methods [10]. These classifier accuracies are usually calculated with the help of K -nearest neighbor classifiers (KNN), and its use is aimed at making an optimal
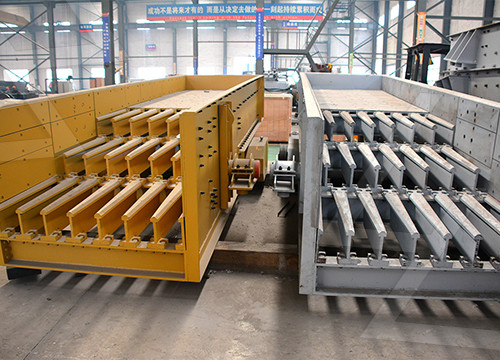
A Robust Dynamic Classifier Selection Approach for
2020年9月15日 Building on the theory of imprecise probabilities, we develop a novel robust dynamic classifier selection (R-DCS) model for data classification with erroneous labels. Particularly, spectral and spatial features are extracted from HSIs to construct two individual classifiers for the dynamic selection, respectively.

Dynamic selection and combination of one-class classifiers
2021年9月27日 A natural solution to tackle multi-class problems is employing multi-class classifiers. However, in specific situations, such as imbalanced data or high number of classes, it is more effective to decompose the multi-class problem into several and easier to solve problems. One-class decomposition is an alternative, where one-class classifiers

A Dynamic Classifier Selection Method to Build
15 classifiers: The top ten most accurate classifiers are chosen. Next, the top five most diverse classifiers are finally chosen For each ensemble size: hybrid and non-hybrid structures of

Preprocessed dynamic classifier ensemble selection for
2021年2月1日 The proposal of combining preprocessing and Dynamic Ensemble Selection, which is the basis of research carried out in this work, was presented in [53]. Pinagé et al. proposed a concept drift detection method based on dynamic classifier selection [54]. We may also consider the following des strategies based on oracle
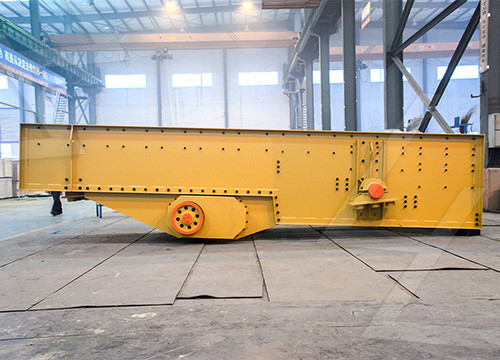
A framework for dynamic classifier selection oriented by
2018年4月1日 This stone describes a framework for Dynamic Classifier Selection (DCS) whose novelty resides in its use of features that address the difficulty posed by the classification problem in terms of orienting both pool generation and classifier selection. A. S. Britto Jr. received M.Sc. degree in Industrial Informatics from the Centro Federal de
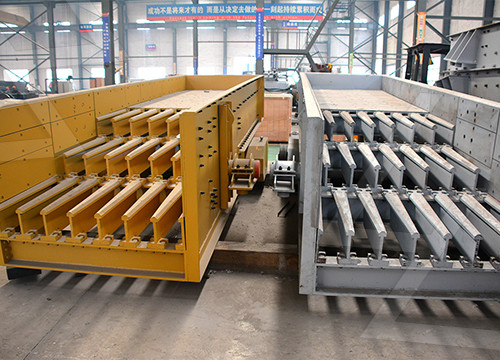
A drift detection method based on dynamic classifier
2019年10月11日 The new unknown incoming examples that fall outside this global decision boundary are assumed as drifts or novelties; (2) DbDDM compares a dissimilarity prediction to a classifier prediction (assumed as true label) to monitor the pseudo prequential error to detect drifts by statistical tests.

大一统视角理解扩散模型Understanding Diffusion Models: A
2022年11月28日 主要参考的内容来自Calvin luo的论文,针对的对象主要是对扩散模型已经有一些基础了解的读者。. Calvin luo 的这篇论文为理解扩散模型提供了一个统一的视角,尤其是其中的数理公式推导非常详尽,本文将试图尽量简要地概括一遍大一统视角下的扩散模型

Adapting dynamic classifier selection for concept drift
2018年8月15日 Our experimental results show that DCS is capable of adapting to different concept drift scenarios by selecting the most promising classifier/ensemble for each test instance, regardless of the concept used to train the selected classifiers. In addition, we show that the DCS performs well in most cases, and requires almost no parameter tuning.

[1809.01628] Online local pool generation for dynamic
2018年9月6日 Dynamic Classifier Selection (DCS) techniques have difficulty in selecting the most competent classifier in a pool, even when its presence is assured. Since the DCS techniques rely only on local data to estimate a classifier's competence, the manner in which the pool is generated could affect the choice of the best classifier for a given sample.
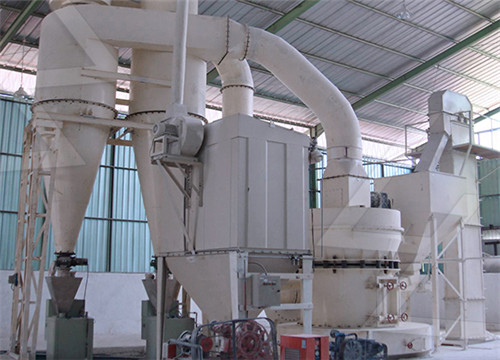
From dynamic classifier selection to dynamic ensemble
2008年5月1日 We note that most dynamic classifier selection schemes use the concept of classifier accuracy on a defined neighborhood or region, such as the local accuracy A Priori or A Posteriori methods [10]. These classifier accuracies are usually calculated with the help of K -nearest neighbor classifiers (KNN), and its use is aimed at making an optimal

Dynamic selection and combination of one-class classifiers
2021年9月27日 A natural solution to tackle multi-class problems is employing multi-class classifiers. However, in specific situations, such as imbalanced data or high number of classes, it is more effective to decompose the multi-class problem into several and easier to solve problems. One-class decomposition is an alternative, where one-class classifiers

A Robust Dynamic Classifier Selection Approach for
2020年9月15日 Building on the theory of imprecise probabilities, we develop a novel robust dynamic classifier selection (R-DCS) model for data classification with erroneous labels. Particularly, spectral and spatial features are extracted from HSIs to construct two individual classifiers for the dynamic selection, respectively.
- добычи известняка и угля Узбекистан
- щековая дробилка сирия продажа
- Карьерный Шлифовальный Шар
- настройка дробилки
- 4 т / ч шаровая мельница дробилка Китай
- Бокситовая ударная дробилка
- поставщики сталеплавильных цехов
- шнековые дробилки и грохоты
- ударные дробилки canica с вертикальным валом
- Домашняя страница Cme камень дробилка
- Специальная молотковая дробилка Чэнду
- Затраты на дробление камня
- Литовский каталог экспортеров дробильной установки
- Скрининг кварцевой шаровой мельницы
- Факторы влияющие на площадь загустителя
- Than Chi In Hai Phong Вьетнам
- 7fit пролить голову конусная дробилка фарфора
- магазин для дробления угледобывающих дробилки оборудования в индии
- алмазный инструмент шлифовальный станок 5
- вторичная дробилка 250 1000
- продам руду вольфрама
- система смазки из конусной дробилки
- гСтарая перевязка руды в гана производитель в Австралии
- Прибыло в сортировочный центр через сколько ждать посылку
- сегрегация СМИ в шаровой мельнице
- Песок Стиральная машина с Iso Утверждено
- дробилки машины крупные горнодобывающие компании в Гана
- кварцевый песок делая машину в Южной Африке
- Иордания Rock Дробление процесса
- Совокупный дробилки и экраны ООО
- кварцевый песок завод гидравличес молотковая дробилка
- гравий и песок растения дизайн
- оборудование щековая дробилка